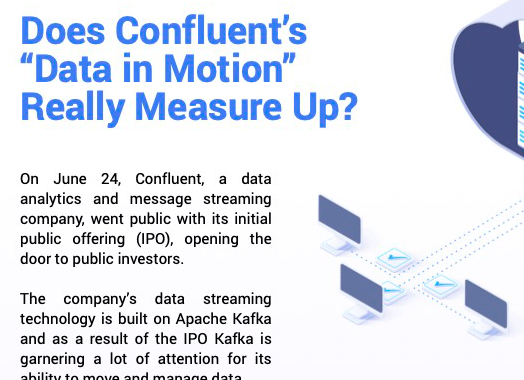
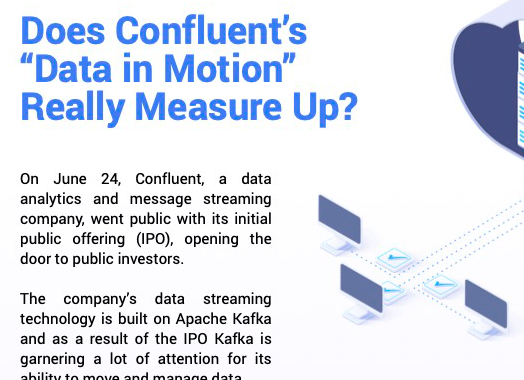
Does Confluent’s “Data in Motion” Really Measure Up?
On June 24, Confluent, a data analytics and message streaming company, went public with its initial public offering (IPO), opening the door to public investors.
The company’s data streaming technology is built on Apache Kafka and as a result of the IPO Kafka is garnering a lot of attention for its ability to move and manage data, with Confluent even adopting the tagline “Data in Motion,” in its recent marketing efforts.
But what is “data in motion,” and if your business is looking to make data-driven business decisions and incorporate artificial intelligence (AI) and machine learning (ML) into your operations, is Confluent Kafka right for you?
Well, if you need multi-tenancy, true tiered storage, low latency and zero data loss (and a range of other important features)—especially, if you’re a large enterprise with a lot of critical data flowing through your business—the answer is probably not.
Why? Because Apache Pulsar, powered by Pandio, outperforms Confluent’s Kafka on every level.
The Confluent-Kafka Connection
In Confluent’s Founders Letter, filed within the company’s S-1 Registration Statement with the United States Securities and Exchange Commission (SEC), CEO and co-founder Jay Kreps explains that Kafka’s origins go back to his days at LinkedIn, where in 2011 he and Jun Rao and Neha Narkhede (who would go on to join Kreps to found Confluence in 2014) helped build Kafka as a single solution to unites data from a variety of technologies into a single platform.
In Confluent’s S-1, the company explains that Kafka’s earliest days were tied to LinkedIn and it was used to process billions of the company’s messages and data streams.
“Kafka was built to be open source, and we wanted it to do much more than serve one use case in one company,” Kreps said.
Eventually, Kafka became part of the Apache Software Foundation, extending the open-source technology to a growing number of users worldwide.
Over the years, Kafka has undergone a range of evolutions and changes, including the development of data infrastructure that supports real-time data streams and links together all of a company’s systems, data, and applications into a single solution.
Data in Motion: What Does That Actually Mean for Your Business?
Traditionally, database technologies have primarily handled data at rest, meaning it’s not data that’s moving from one system or device to another. It’s data like the stuff that’s stored on your computer, drive, or server.
Data in motion is data that actively moves from one location (for example an application, system, or device) to another and usually moves through an internet connection or private network connection.
A simple way to think of this is storing data in the cloud. You may have data that lives on your smartphone or computer, but to back it up, you may have scheduled or on-going movement of that data into your cloud storage.
But when it comes to small and mid-sized businesses (SMBs) and large enterprises, these data transits increase exponentially, and today, with an increasing number of systems, devices, and applications needed to run operations, the volume of data in motion across most organizations just keeps increasing.
You’ll often hear industry buzz words like “digital transformation” when companies talk about moving data in and out of the organization, especially into the cloud. Confluence’s S-1 hits on this when it talks about the need for modern business to take a digital-first approach to their operations.
Unfortunately, many businesses see digital transformation or the move to the cloud as having to ditch old infrastructure and systems to rebuild new architecture that better supports new technology. Instead, your data streaming platform should enable you to improve your operational processes by integrating your existing systems and applications and sharing otherwise disparate data in a way that you can put it to use for your business.
And while leveraging data in motion is important, it isn’t the core driver for business. What your business really needs is the ability to query and move data (that is truly a single source of truth) to AI and ML applications, which can synthesize and analyze that data, leading to data-driven decisions.
It’s not all about the fact that your data is in motion; it’s about learning from your data and using it to make your business stronger today and for scalability in the future.
So where should your focus be? It’s all about how you connect all the systems, applications, and data within your organization, how that data is processed, and what happens once you move it into AI and ML models.
No platform on the planet does this better than Apache Pulsar.
Let’s take a closer look at some of the touted features of Kafka and look at them closer from a Pulsar perspective
Multi-Tenancy
In data technologies, the term multi-tenancy describes how a single instance in a software can be used for multiple user groups. For example, the infrastructure within a cloud might be shared between a range of organizations or users but with security limits that prevent clients within the same multi-tenant environment from accessing each other’s data.
What’s a tenant? A tenant represents a unit within the shared environment, for example, applications, software, or teams.
Examples of some common multi-tenancy platforms today are:
- Dropbox
- Google Apps
- Salesforce
- Microsoft 365
Conversely, single tenant describes software that serves just one client, for example, data stored on a server with dedicated access and infrastructure. While there are some benefits of single tenant applications, for example, data isolation to help secure it from other customers like those in a multi-tenant environment, most modern operations today need more diverse data flow options.
Kafka doesn’t support multi-tenancy the same way Apache Pulsar does. For example, Pulsar’s foundation is built on the ability to enable multiple teams or groups to share a single cluster simply by using different access controls or different namespaces. Kafka can’t do that. The Kafka alternative is to create new clusters for your additional teams. That ends up costing you more money and using up more of your resources.
Pulsar’s multi-tenant features also mean you can process data requests faster and with higher throughput, less latency, and practically zero data loss. That means you can scale much faster with Pulsar than you can with Confluence.
You don’t have to do a lot of online searches to see how many people are taking the Kafka multi-tenancy claim to task. Want to know more? Contact a Pandio representative today and we’ll help explain it in greater detail.
Geo-Replication
Geo-replication is a way to back up your company’s data to ensure you still have access to that data if one or more data centers goes down. In geo-replications, the data is distributed across different networks to different geographical locations.
For large enterprises, data backups can take up a lot of space, so when you’re looking for data storage options, you need to ensure the storage provider can provide enough space to handle all of the data and grow with it as your company scales. That could mean managing multiple locations and data centers and ensuring each is accurate and up-to-date, ultimately decreasing downtime should you experience a disruption.
Apache Pulsar does this natively, whereas you’ll need a workaround or add-on for Confluence’s Kafka alternative. That’s because in Kafka, your data is organized into clusters and those clusters are then distributed among multiple data centers. When you need to copy your data across multiple data centers, you’ll need to use a tool such as MirrorMaker. Not only is it time consuming, it’s also complicated and doesn’t always work as expected.
Real geo-replication in Pandio is way more flexible. Instead of employing an additional tool, you can set up both synchronous and asynchronous data replication right in the platform.
Tiered storage
When we’re talking about large companies—or even smaller companies today that will scale in the future—storage (space and cost) is a huge issue. The more data you have, the more data back-ups you need, and traditionally, the more money you’ll spend.
Kafka doesn’t separate your data compute from your data storage, which can complicate data restoration, make it more expensive, and even create some compliance challenges. If you want to keep old data for a long period of time, you may have to maintain that storage yourself. In traditional data storage environments like this, some companies have had to make the difficult decision to let some of its older data go altogether—to save space and cut costs. That’s because streaming platforms like Kafka don’t have true tiered storage.
With tiered storage you should be able to segment all of your data and then make storing decisions based on data relevance, quality, and integrity. That’s because not all of your data is the same and not all of your data is needed for your most critical operations.
Apache Pulsar’s tiered storage is a game changer. It enables you to store as much data as you need to—even going back months or years—which can be important for data scientists looking to employ machine learning on your data to help you make better business decisions.
Pulsar’s tiered storage option also saves you money, ensuring you’re always paying the right amount of money for the right amount of storage you need, regardless of how your business changes or grows.
Three in One Messaging
When it comes to distributed messaging patterns, you don’t want to be stuck with only one option, and if you’re using Confluent, you’ll essentially work with event streaming only. To get Kafka to queue, you’ll need a separate tool, RabbitMQ.
Apache Pulsar, however, natively supports streaming, messaging queuing, and pub-sub. It uses server-to-server messaging and was developed on a pub-sub pattern. You can use this one platform to event stream, process streams, as a data pipeline, and for microservices.
Zero Data Loss
When you’re moving millions, billions, or trillions of data sets across your message streaming platform, zero message loss might seem like a pipe dream, but with Apache Pulsar, it’s 100% reality.
Data loss is an issue that plagues many organizations. You’ve probably been there when a coworker—or maybe even yourself—accidentally deleted a file and you then found out it was erased before the latest backup and now it’s gone gone. Ouch. What a nightmare.
Unfortunately, Kafka has known issues with data loss and sometimes data that should have been delivered through the system just didn’t end up where it needed to be or other times there are quantity or quality issues for that data.
While some teams using Kafka have come up with their own innovative ways to work around this potential data loss issue, ensuring data integrity is foundational in Apache Pulsar. In fact, Pulsar was built from the ground up to tackle data loss issues and it can operate much faster than Kafka. One engineer even did a test where he attempted to force message loss on Apache Pulsar clusters by killing off nodes to slow the network and create packet loss. Pulsar had no missing or out-of-order message after being put through several diverse scenarios. Similar tests against Kafka successfully discovered message failures in Kafka.
Pulsar vs Kafka
While maintaining real-time data in motion is important, to do it right—and to get the most out of your data—you need a robust architecture that supports multiple messaging patterns, supports multi-tenancy, has reliable and easy-to-set up geo-replication options, tiered storage, and ensures your data is available when you need it, both in quality and quantity. When you look closer under the hood, you’ll quickly see the Confluent’s Kafka will always come up short when paced against Apache Pulsar.
If you want to own your data in motion and put it to work for you, try Pandio’s Pulsar for free and see why a growing number of companies now rely on Apache Pulsar as its only messaging solution. You’ll get three times better throughput, be able to scale three times faster, and improve your cost efficiencies by 40% or more. Have other questions or want to know more about how Pulsar outperforms Kafka at every turn? Contact us today and we’ll be glad to show you more.